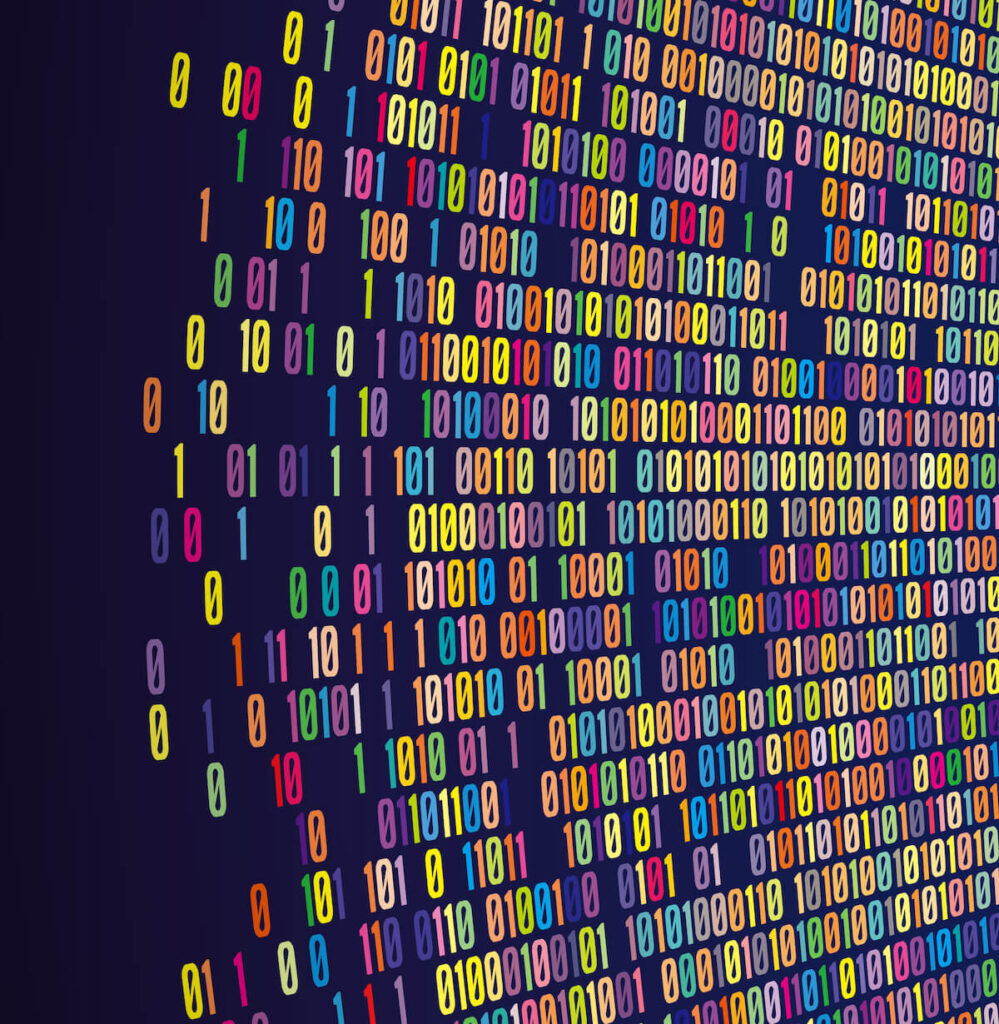
In emerging economies, the growth of mobile phone use has contributed to an explosion of data that can be fed into algorithms to make predictions about individuals, including who is creditworthy, and offer financial products and services. As of 2020, half of the world’s population used mobile internet and digital finance offerings have grown in tandem, often framed as engines of financial inclusion. The massive growth in available data and companies to serve customers that had previously been invisible to the financial system might suggest that the problem of thin-file customers has been solved and long-standing financial inclusion gaps are closing.
But the truth is, we don’t know what the impact is yet.
So amidst the rush to generate and leverage new data trails, CFI is embarking on a learning agenda around financial inclusion at the data margins. In pursuit of that, we are rethinking assumptions, and learning from broader tech-oriented conversations about the intersection of alternative data, algorithms and digital profiling, and how these more technical issues interact with socio-economic and gender-oriented issues surrounding digital literacy, financial capabilities, and social norms.
What Does it Mean to Lend to a Phone Line?
In the context of financial inclusion, making people visible to the financial market nowadays increasingly means having a digital financial identity based on volumes of (mobile) data that’s both related and unrelated to the actual financial behaviors of an individual. The expansion of digital lending, for example, utilizes non-traditional mobile data such as SMS, device usage and model information, social network activity, contact lists, call logs, app use, location data, and more to construct a picture of an individual’s cash flow or income.
Some of these sources are intuitively linked to financial activity, such as SMS receipts for mobile wallet transactions. Other data — referred to by some as “fringe” alternative data — like social media activity, is further removed and its value as a fair and accurate predictor of creditworthiness is less established.
Given that data-driven models leverage phone-specific variables, the limits on women’s phone use or migrants’ access to a SIM card will change the data trail they leave and, in turn, the decision that an algorithm may make about their access to a loan.
The limits on women’s phone use or migrants’ access to a SIM card may change the decision an algorithm makes about their access to a loan.
In developing economies, women are less likely to own a phone and access mobile internet. Phone-sharing commonly acts as a barrier to phone ownership for women as well as other vulnerable groups like migrants or refugees. It’s prevalent due to cost constraints or gaps in identity documents required for registering a SIM card. What does it mean when an algorithm is facilitating lending to a phone line, rather than an individual? What are the implications of this and other extant barriers in determining how inclusive these new tools are?
Risks of Data Marginalization: Where to Begin?
In the U.S. and Europe, risks and evidence of data marginalization have prompted calls for greater oversight, but little research has documented what these risks look like in the data environments of developing markets. There is much that we need to learn:
- How large is the risk of cementing exclusion in a data-driven financial system for consumers at the data margins?
- What strategies and tools are available to companies, investors, and regulators to address these risks, and what resources and skills do they require to be effective?
- What are the incentives for private sector companies to act on this risk?
- Globally, we see strides made on data privacy and emerging digital rights, but how will people with limited digital capability be able to meet these new rights and responsibilities?
CFI and others, like our friends at Women’s World Banking, are beginning to address these questions and establish a learning agenda for the sector.
It is critical we set out on a path for answers now in this period of global data ecosystem transformation. As a fintech from Southeast Asia explained, “You can’t make those [anti-bias] changes when you’re doing a million loans a day… a little bit of a loss now and a gain later is better than taking a loss at that scale.”
Let this remark serve as a wake-up call for all development stakeholders that are building data solutions today not to lose sight of the people behind the mobile phones and to examine how technology and data can help us build an inclusive tomorrow.
This topic is one of CFI’s strategic pillars, so consider this the first mile of a learning journey that will take all of us to a better understanding of data risks and opportunities as they relate to financial inclusion. Stay tuned to this space, sign up for CFI email updates, and follow us on social media for coming explorations of data risks and opportunities and how they affect low-income consumers.